11 Kellogg Insight Nor can managers rely on data scientists to take the lead. Ultimately, it is the man - ager’s job to choose which problems need to be solved and how the company should incorporate analytics into its operations. Executives, after all, are the ones who have to make decisions; therefore, they should play a central role in determining what to measure and what the numbers mean to the company’s overall strategy. Understand the Data- Generation Process “There is a view out there that because analytics is based on data science, it somehow represents disembodied truth,” Zettelmeyer says. “Regrettably, that is just wrong.” So how can leaders learn to distinguish between good and bad analytics or AI? “It all starts with understanding the data-generation process,” Zettelmeyer says. “You cannot judge the quality of the analytics if you don’t have a very clear idea of where the data came from.” Zettelmeyer says most managers share a common behavioral bias: when results Based on insights from Florian Zettelmeyer Analytics vs. Artificial Intelligence Let’s say an advertiser is bid- ding to show an online ad to a customer. They might use mass-scale experimentation to determine how effective an ad will be for certain types of customers and then create a model that automatically scores every potential target in terms of their likelihood of responding in order to calculate a bid. The model itself would be developed and updated offline. But the actual scoring and bidding could be done in real time. “It’s this crazy combination of using mass-scale experimen- tation, using very sophisti- cated machine learning, and using automation in order to create something that, if per - formed by humans, would be considered intelligent,” says Zettelmeyer. “The future of learning in marketing is going to come from some form of marrying these together.”
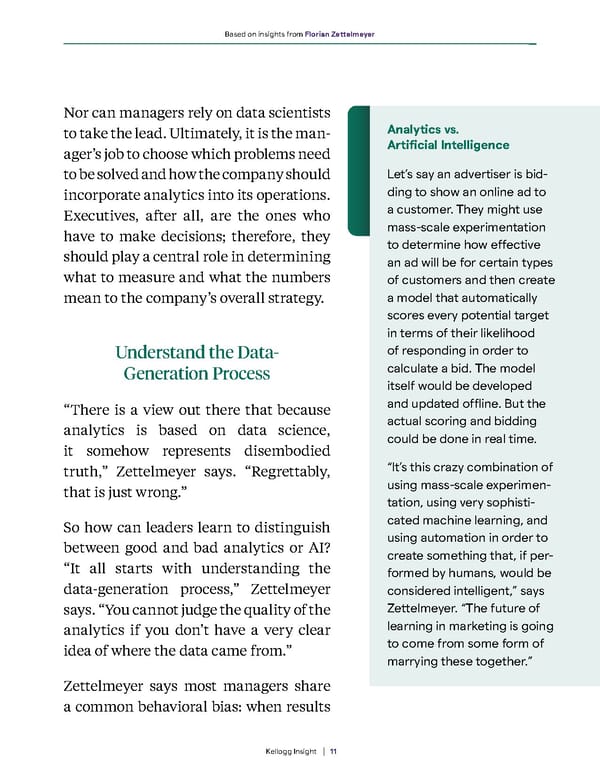