114 Kellogg Insight they need to improve their algorithms. Take a straightforward example, such as knowing which coupons to send to which customers, when, and in which format. Instead of collecting a lot of information about a given customer from a range of sources, the retailer might generate numerous synthetic customers from the bits of information they do have and play out a number of scenarios to deter - mine which coupon is most effective. Synthetic customers still require some real data to generate, however. While long-standing companies like Sears—which have decades of data from loyalty programs and customer credit cards—are well positioned to build composite shoppers, smaller players might struggle. But companies that can figure it out stand to reap huge benefits. “You have unlimited and infinite customers upon which to test,” Pah explains. “That, I think is, brilliant.” Understanding that Data Is the Product The increasing value of data is also encouraging startups to consider whether their products can do double duty: fill an immediate need for customers, but also create datasets that can be licensed to more estab - lished companies. “People are really realizing the importance of data as a product,” says Pah. Take Netradyne, a startup that uses AI to monitor whether drivers of large commercial fleets are operating safely. Its image recognition capabilities Based on insights from Adam Pah
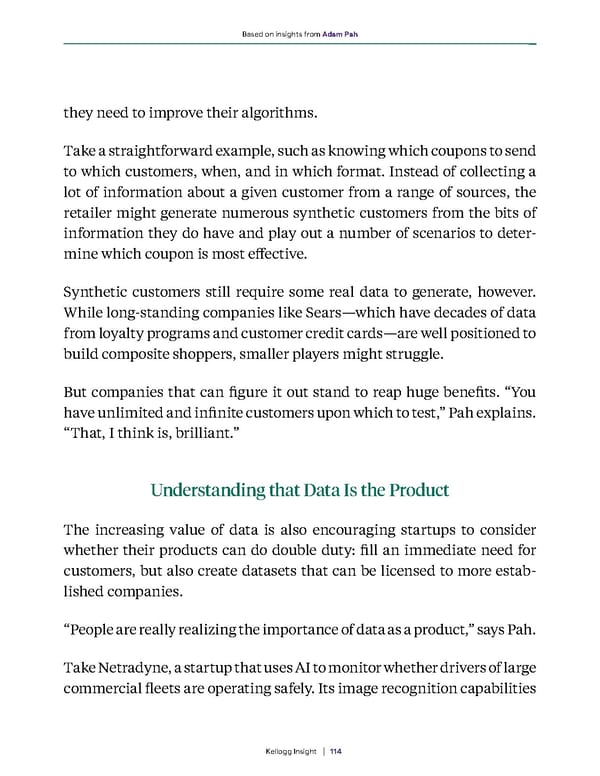