13 Kellogg Insight or was the consumer already interested? “Algorithms are limited by the data you feed them,” says Zettelmeyer. “If opportunistic data created in the normal course of business are polluted, what you can learn is very limited.” This is not just a marketing problem. Take, for example, a hospital that wants to replace its ultrasound machines. Thanks to advanced wireless sensors, the hospital is able to measure in the course of business exactly how long it takes to perform an exam using the new devices, a metric that would help it decide whether to switch over for good. But the data show a surprising result: the new device is taking longer to use than the older one. What the hospital had not accounted for was a preexisting difference between two groups of technicians: novice technicians and experienced technicians. It turns out that more novice technicians, who were naturally slower than the experienced ones, were choosing to use the newer device, and this skewed the data. “The problem,” Zettelmeyer says, “is one of confounding technician experience with the speed of the device.” Again, analytics failed because it overlooked fundamental questions: What makes technicians choose one machine over the other? Is everything about the usage of the two machines comparable? And if not, was the correct analytics used to cor - rect for that? Understanding the data-generation process can also uncover the prob - lem of reverse causality. Here, Zettelmeyer points to the case of a com - pany deciding whether or not to limit promotional emails. The data reveal that promotional emails are extremely effective: the more emails a customer receives, the more purchases they are likely to make. But what is not apparent in the data is that the company is following a piece Based on insights from Florian Zettelmeyer
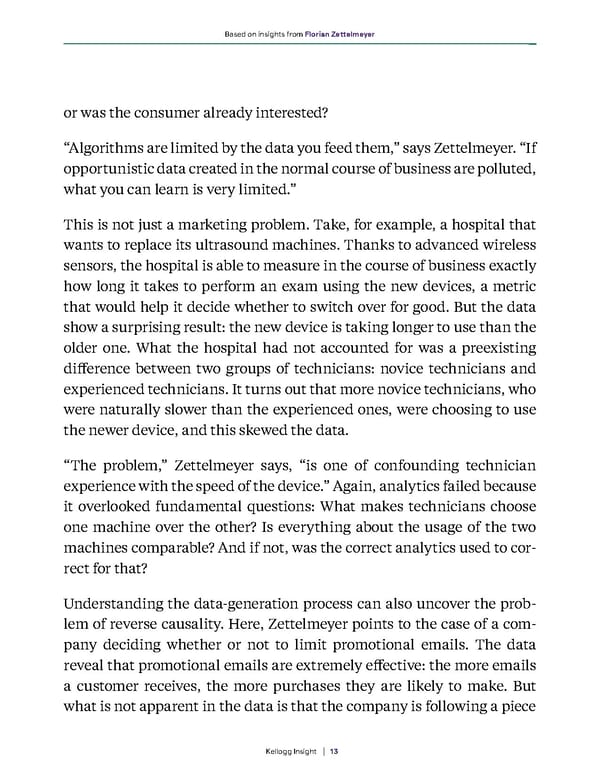