100 Kellogg Insight analysts extract useful information from these reviews? And second, could machine-learning algorithms enable them to do so more efficiently? Mining Product Reviews To address this first question, the researchers brought in a marketing-con - sulting company called Applied Marketing Science, Inc. (AMS). AMS has over twenty years of experience in market research and customer-need elicitation, and they had recently conducted a for-client interview-based study of customer needs for oral-care products. “It was very convenient from both a business and a research perspec - tive,” Timoshenko explains, as toothbrushes represent a fairly standard product category and one with plentiful Amazon reviews. Moreover, AMS was excited about the researchers’ questions, and the company was eager to partner. When it comes to oral-care products, many customers report needs that are fairly straightforward: the products need to keep their teeth clean and white, keep their gums healthy, and not damage any previous den - tal work. But other customers might mention less expected needs, such as knowing how long to spend on various parts of their mouth during their oral-care routine. This might lead to product ideas, such as tooth - brushes that beep at timed intervals or shut down after a certain number of minutes. The experiential interviews conducted by AMS revealed 86 different cus- tomer needs for oral-care products, a typical number for such a product category. The goal of analyzing these customer needs is to find a hidden Based on research from Artem Timoshenko
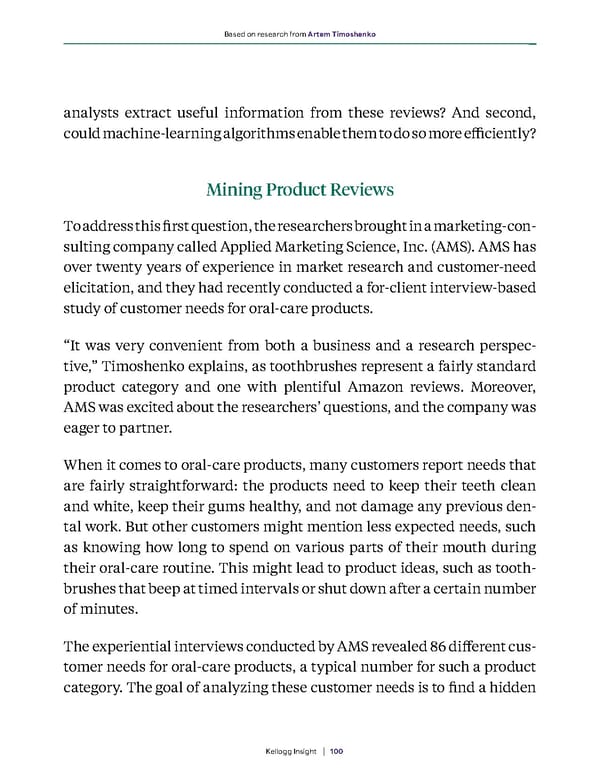