71 Kellogg Insight great at analytics. Then one day the models stop working. Mistakes that fueled the models are now gone and the analytic models are starved.” The paradox is that the better the firm gets at gleaning insights from analytics—and acting on those insights—the more stream - lined their operations become. This in turn makes the data result - ing from those operations more homogeneous. But over time, homogeneity becomes a problem: variable data—and, yes, mistakes— allow algorithms to continue to learn and optimize. As the variabil - ity in the new data shrinks, the algorithms don’t have much to work with anymore. The paradox leads to a rather startling recommendation: “Occasionally, you need to purposely mess stuff up,” Florian Zettelmeyer says. “You design variation into your data in order to be able to derive long-run insight,” explains Zettelmeyer, also a professor of marketing at Kellogg. Zettelmeyer and Anderson are academic directors of Kellogg’s Executive Education program on Leading with Big Data and Analytics; they are also writing a book about data science for leaders. Here, they offer a look at how the best firms have found a way to sidestep the Analytics Paradox. From Optimization to Stagnation In some sense, the value in big data lies in its messiness—in the often unexpected variation in how events play out and the myriad ways these events help establish connections between variables that can help peo - ple make better decisions. Based on insights from Eric T. Anderson and Florian Zettelmeyer
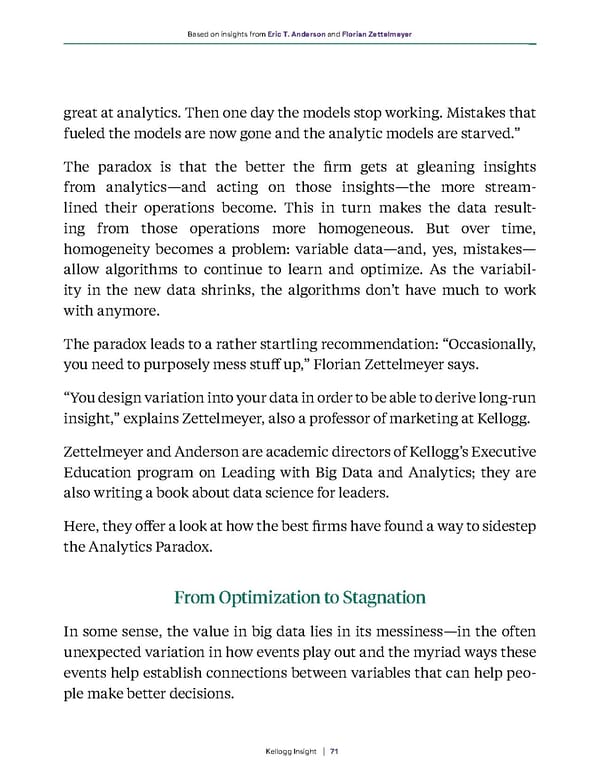