78 Kellogg Insight all, the data might have been entered incorrectly or appear as the result of a modeling error. Or it may represent a freak accident—a set of circum - stances unlikely to replicate itself. Why waste time accounting for the easily discountable? Resist that urge, Shapiro says. It is always worth examining why the out - lier occurred. Shapiro comes to this insight firsthand. While studying the effective - ness of Spanish language instruction in West Virginia’s middle schools, he found that overall, students who received online instruction scored about the same as those who received it in person. But he also found that face-to-face class instruction produced a wider range of experiences. Investigating further, Shapiro learned that most of the high-performing in-person students came from one specific class. After he had presented his results, the state’s board of education advised him to exclude that class from analysis because its instructor was known to work well with students. “They said she was the best thing that’s ever happened to the state of West Virginia from an education perspective,” Shapiro says. But Shapiro realized that, rather than excluding this instructor’s stu - dents, the board of education should do the exact opposite. They should find out what this person was doing and try to replicate it. What this outlier ultimately demonstrated was that, under the right circumstances, the in-person classroom model had the potential to outperform online instruction. This is an important first step toward understanding the potential of these outliers, Shapiro says. “They Based on insights from Joel K. Shapiro
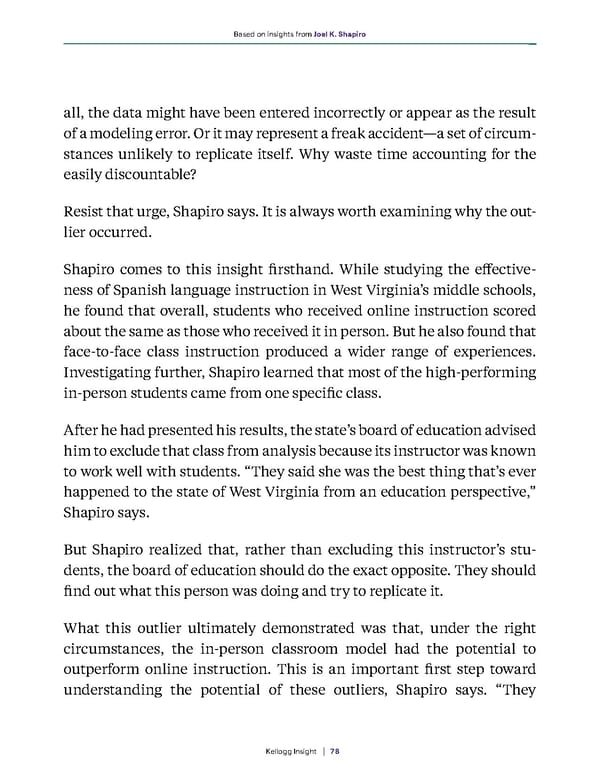