21 Kellogg Insight making decisions about whether or not to give somebody a mortgage, you might be able to use historical data and train up the deep-learning system, and that will give you a yay or a nay. But when you ask that sys- tem, ‘Why did you just deny someone a mortgage?’ the system can’t tell you. You’ve got to ask yourself if that is okay.” Questions about whether a particular technology is appropriate for a problem aren’t hypothetical; they can have real—and really powerful— implications for organizations, their customers, and their employees. For example, Amazon used deep learning to train a resume-filtering sys- tem on data that included the original resumes of staff members, as well as their subsequent performance reviews. The company’s hope was that the filtering system would pick up on signals—particular skills or expe - riences—that would indicate future success at the company. But when they started to feed resumes through their system, they noticed a problem. “It turned out the system was gender biased,” says Hammond. The system had picked up on sig - nals Amazon never intended it to learn from, and it learned to penalize female candidates. But, Hammond stresses, it wasn’t the actual algorithm that was biased— it was Amazon itself. Because the company used data from perfor - mance reviews to train the sys- tem, and because women similarly Learn more about how bias creeps into AI when businesses aren’t looking. Based on insights from Kris Hammond
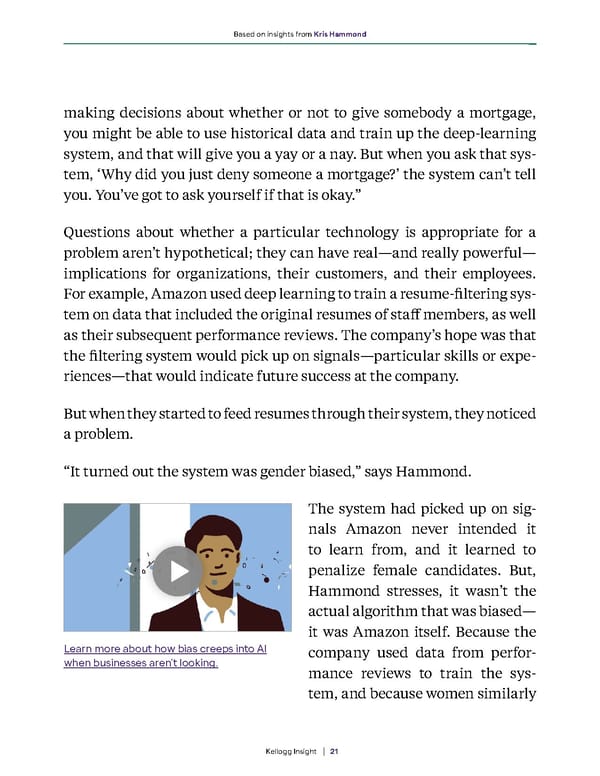