20 Kellogg Insight uniquely equipped to handle? Perhaps. But Hammond argues that the level of knowledge required to make smart decisions about AI is different than one might imagine. He offers up cellular technology as a metaphor. Most of us have never heard of cell-signal encoding; fewer could pass a test on how antennas work. Yet we’re more than comfortable using our cell phones: we know all the things they can do for us, as well as the practical limitations on their utility, such as the fact that they will die unless we occasionally charge them. What does this look like in the context of AI? Well, executives do not need to understand the algorithms that underlie gradient descent or backpropogation. But they might benefit from what Hammond calls a functional understanding of some of the most popular AI technologies. So they should understand that a common technique called “deep learn - ing” is extraordinarily powerful when it comes to recognizing patterns, for instance, but that it has some important constraints as well. Notably, its algorithms require a lot of data to train—hundreds of thousands of examples. And deep learning is opaque: once it has learned to recognize patterns, it cannot tell you what features it is picking up on or empha - sizing as it recognizes and categorizes. That means that, from a busi - ness perspective, it shouldn’t be used in those places where the ability to explain or audit decisions is required. It’s this level of information that should influence decisions about whether a piece of technology might be appropriate for a given task. “So you look at that and ask, ‘What does that mean, from a functional point of view, in terms of my business?’” says Hammond. “Well, if you’re Based on insights from Kris Hammond
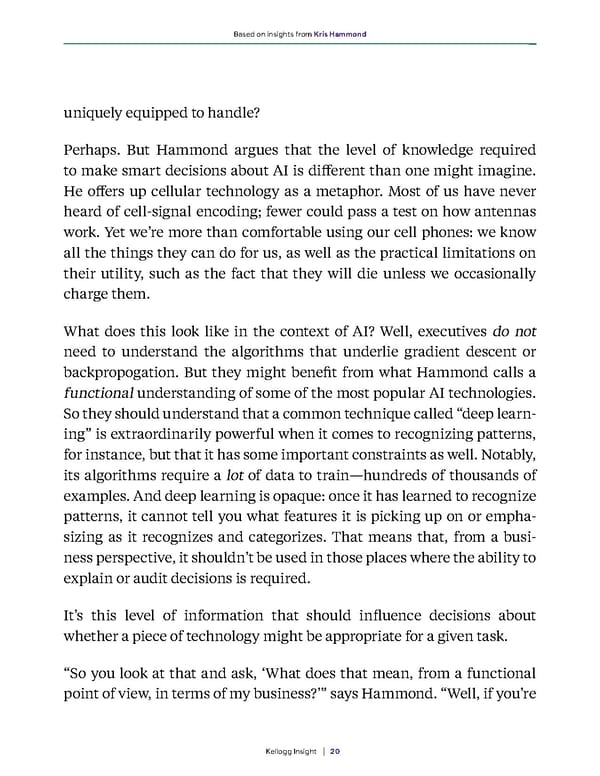