121 Kellogg Insight ZETTELMEYER: Okay, accountability. What’s your second focus area to build trust in AI? SUH: It’s a focus on values. What are the norms for a common set of core principles that you operate under? And depending on different cultural norms, whom do you bring into the process [of creating these principles]? There’s a third focus area around data rights and data privacy, mostly in terms of consumer protection—because there are companies that offer an exchange of data for a free service of some sort, and the consumer might not realize that they’re actually giving permission, not just for that one instance, but for perpetuity. ZETTELMEYER: Do you think it is realistic today to think of consumers still having some degree of ownership over their data? SUH: I do think there’s a way to solve for this. I don’t think we’ve solved it yet, but I do think there’s a possibility of enabling individuals to under - stand what information is being used by whom and when. Part of that is a burden on the institutions around explainability. That’s number four—being able to explain your algorithm: explain the data sets that were used, explain the approach holistically, be able to detect where you might have biases. This is why explainability and fairness—that’s number five—go hand in hand. ZETTELMEYER: In an academic context, I refer to this as transparency of execution. I actually thought you were going to say something slightly different, that we need to move to a place where some of the more flexible algorithms like neural networks or deep learning can be interpreted. Based on insights from Florian Zettelmeyer and Inhi Cho Suh
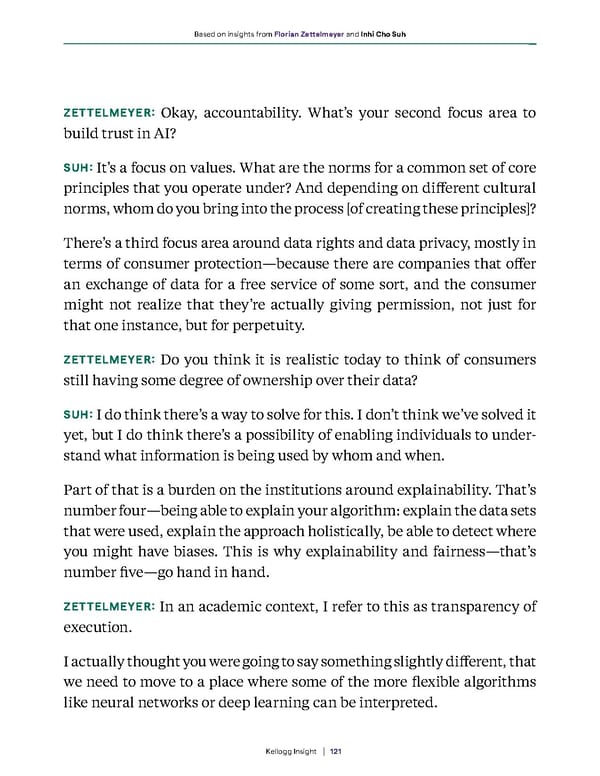