122 Kellogg Insight It’s a hard problem because, in some sense, precisely what makes these algorithms work so well is what makes them so hard to explain. In other words, the problem with these algorithms isn’t that you can’t write them down. You can always write them down. The problem is that it’s very dif - ficult to create some easily understandable association between inputs and outputs, because everything depends on everything else. But I think the point you were making is: okay, even if we do have a so-called “black box” algorithm, a lot of the biases arise, not necessarily from the algorithm per se, but from the fact that we’re applying this algo - rithm to a particular setting and data set, yet it’s just not clear to people how it’s being implemented. SUH: That’s right. When and for what purpose are we actually applying AI? What are the major sources of that data? And how are we working to, if not eliminate bias, maybe mitigate it? ZETTELMEYER: I think a lot of the trust problems that have occurred in the tech industry—and particularly in advertising—over the last years are directly related to a lack of transparency of that type. I’m always amazed that when you go to the big advertising platforms, and you approach them purely as a consumer, and then you approach them as a client, it feels like you’re dealing with two different universes. As consumer, I’m not sure you have the same sense of exactly what’s happening behind the scenes as you do if you happen to be an advertiser, and you have exposure to all the digital tools that you can use for targeting. I think transparency, the way you’re talking about it, is not particularly well implemented in many tech companies. Based on insights from Florian Zettelmeyer and Inhi Cho Suh
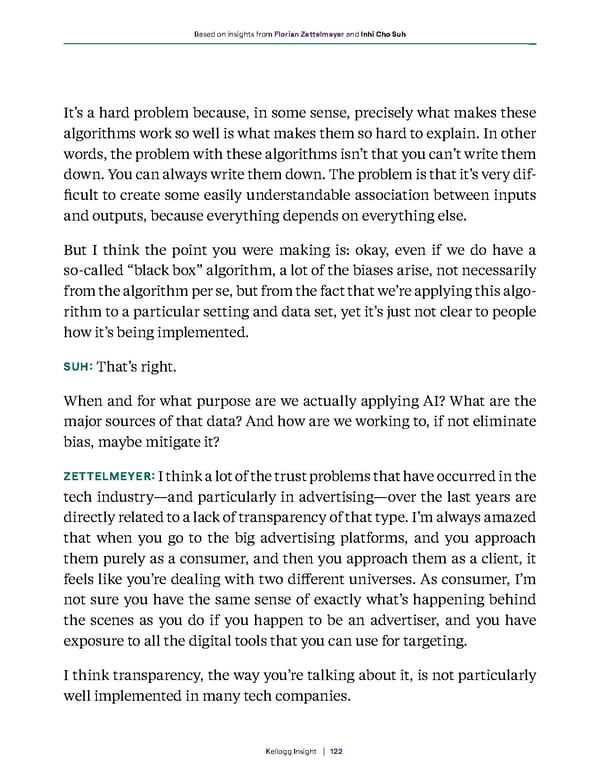