118 Kellogg Insight So therefore you may have loans being arbitrarily denied rather than approved because the lack of sufficient data adds too much uncertainty. ZETTELMEYER: You don’t want to approve a loan unless you have some level of certainty [in the accuracy of your algorithm], but a lack of data doesn’t allow you to make your statistical inputs good enough. What do you think of the Microsoft bot example on Twitter [where the bot quickly mirrored other users’ sexist and racist language]? That’s another source of bias: it seems to be a case where an algorithm gets led astray because the people it is learning from are not very nice. SUH: There are some societal and cultural norms that are more accept - able than others. For each of us as a person, we know and we learn the difference between what is and isn’t acceptable through experience. For an AI system, that’s going to require a tremendous amount of thoughtful training. Otherwise, it won’t pick up on the sarcasm. It’ll pick up on the wrong context in the wrong situation. ZETTELMEYER: That’s right. In some sense, we face this with our children: they live in a world that is full of profanity, but we would like them to not use that language. It’s very difficult. They need a set of value instruc - tions—they can’t just be picking up everything from what’s around them. SUH: Absolutely. And Western culture is very different than Eastern culture, or Middle Eastern culture. So culture must be considered, and the value code [that the algorithm is trained with] has to be intention - ally designed. And you do that by bringing in policymakers, academ - ics, designers, and researchers who understand the user’s values in various contexts. Based on insights from Florian Zettelmeyer and Inhi Cho Suh
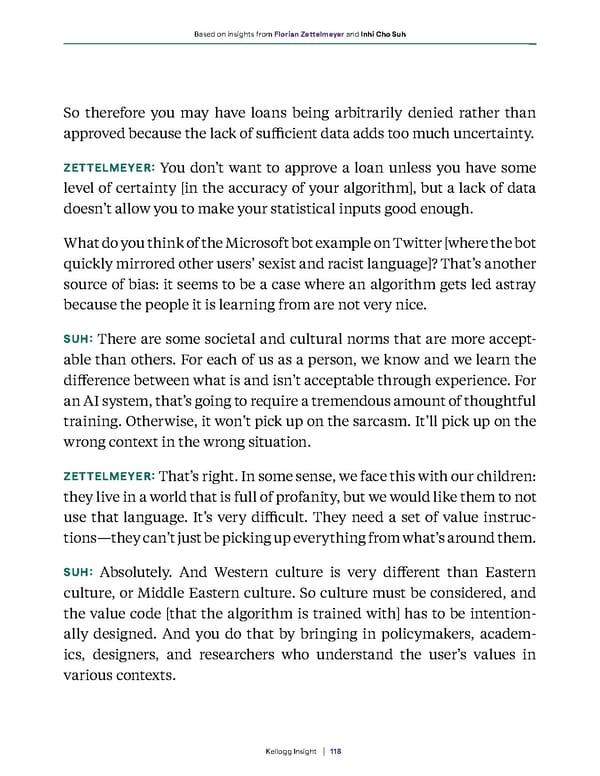