117 Kellogg Insight can be extraordinarily powerful, but they are also “black boxes,” where the inputs and the outputs may be visible, but how exactly the two are related is not transparent. Given the algorithms’ very complexity, bias can creep into their outputs without their designers intending it to, or without them even knowing the bias is there. So perhaps it is unsurprising that many people are wary of the power vested in machine-learning algorithms. Inhi Cho Suh, General Manager, IBM Watson Customer Engagement, and Florian Zettelmeyer, a professor of marketing at Kellogg and chair of the school’s marketing department, are both invested in understanding how deep-learning algorithms can identify, account for, and reduce bias. The pair discuss the social and ethical challenges machine learning poses, as well as the more general question of how developers and com - panies can go about building AI that is transparent, fair, and socially responsible. This interview has been edited for length and clarity. FLORIAN ZETTELMEYER: So, let me kick it off with one example of bias in algorithms, which is the quality of face recognition. The subjects used to train the algorithm are vastly more likely to be nonminority than mem - bers of minorities. So as a result of that, the quality of facial recognition turns out to be better if you happen to look more conventionally Western than if you have some other ethnicity. INHI CHO SUH: Yes, that’s one example of a bias because of a lack of data. Another really good example of this bias is in loan approval. If you look at the financial-services sector, there are fewer women-owned businesses. Based on insights from Florian Zettelmeyer and Inhi Cho Suh
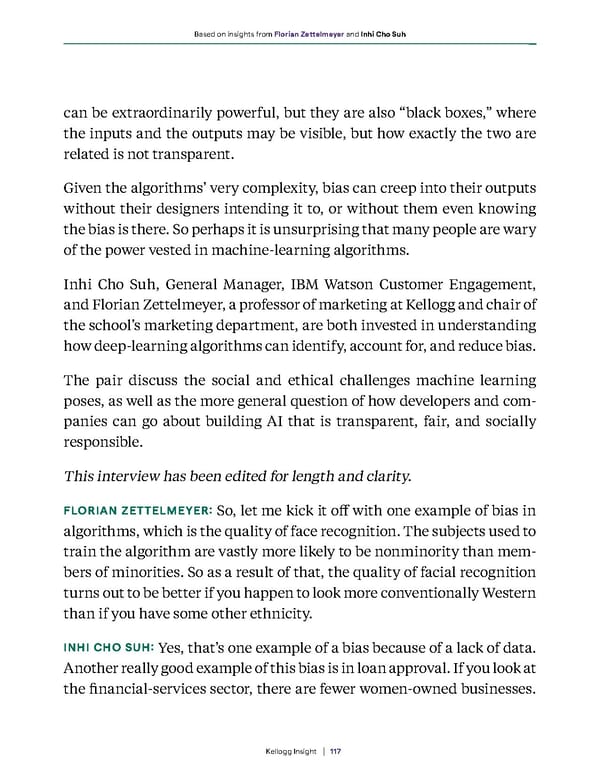