104 Kellogg Insight product innovation. Redundant reviews, also prevalent in the corpus, mention the same deficit or perk over and over again. The researchers found that the prescreening by their algorithm allowed the analysts to find the same number of customer needs in about 20 per - cent fewer sentences. “This was the proof of concept,” says Timoshenko. He is confident that with more experience and engineering, efficiencies would continue to increase, just as the methods for traditional-interview-based market research have improved over years of practice. To that end, the researchers have made their code freely available to companies and are eager to learn about how it is being further developed and applied by companies in different industries. One company in the food industry, for instance, has used the research - ers’ methods and found that they identify very different kinds of customer needs depending on whether they search online reviews or social-media data. Timoshenko says this highlights the fact that, as multiple sources of feedback are considered, the need for machine-learning tools will only grow. “There is even more need for preprocessing this information,” he says, “because there are millions of Amazon reviews for a particular product— but if you want to combine that with the social-media data and online reviews from other sources, it just blows up the amount of content you have to process. And that makes machine learning very important.” Based on research from Artem Timoshenko
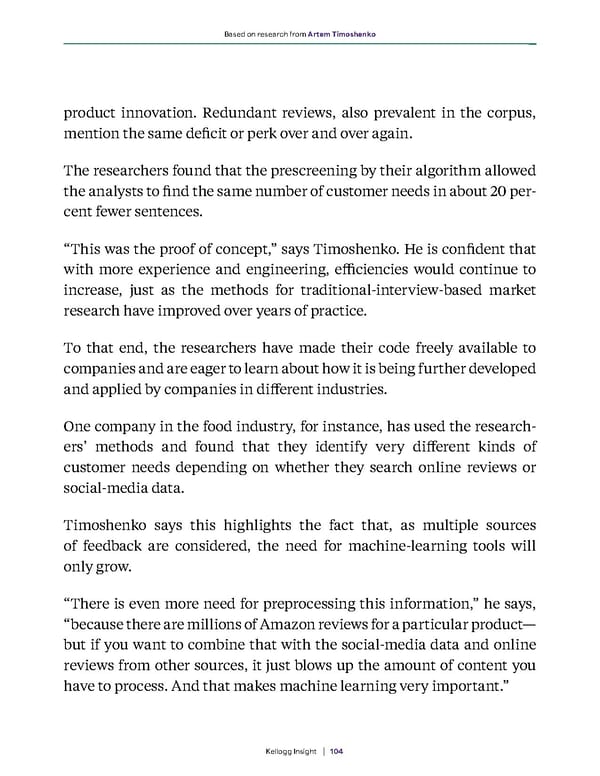