103 Kellogg Insight and focus groups,” says Timoshenko. “And that is the most time-con - suming part of market research for customer needs.” The second finding was that the Amazon reviews contained eight addi - tional customer needs (nearly 10 percent of the total), which were not mentioned during the interviews. These were not materially different from those that were mentioned by customers—they appeared to be just as important to customers and useful for future product development— suggesting that analyzing user-generated reviews could provide a more exhaustive insight into customer needs. Timoshenko suspects that, if additional interviews and focus groups had been conducted, these needs would have eventually emerged. “But doubling the number of interviews you conduct is much more expensive, in money and time, than just doubling the amount of online content we review.” Machines Aiding Humans Next, the researchers attempted to see whether they could use machine learning to make the human analysts more efficient. Specifically, they built an algorithm to “prescreen” the reviews, weeding out less helpful ones so that analysts could make more productive use of their time. The researchers trained an algorithm to prescreen the reviews in two ways: it removed non-informative sentences, and it reduced redundant ones. Non-informative sentences, which make up nearly half of all of the sentences in the corpus, might simply say, “My son loves this pro - duct”—a perfectly legitimate sentiment, but not one that will lead to Based on research from Artem Timoshenko
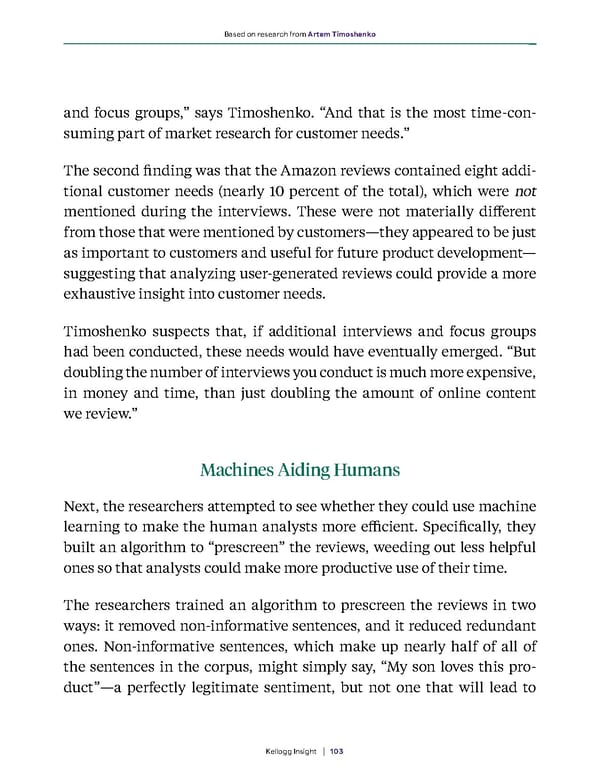