85 Kellogg Insight measurement is being done correctly or not. Someone selling you ads may have incentives that don’t align with yours. In many ways, measurement is very, very simple. To measure anything, you need a control group, where you are either not running the campaign or running it at a much lower volume. And you need that control group to be as similar as possible to the group where you are actually running the campaign. So if someone is telling you about the effectiveness of your campaign, you should be able to ask, “What is the basis of comparison? What was the control and why should I believe that the control is similar to the people who were in the test?” The more similar you can believe those are, the more valid the results are. INSIGHT: That makes sense. But can you explain just how similar a control group needs to be in order to reliably measure whether your campaign is working? On the one hand, there are randomized controlled trials— experiments with true control groups, not unlike ones you would find in academic or medical research. But there also exist a plethora of alterna - tive approaches, workarounds really, such as creating a control group (or market) that is similar in a variety of ways to the one that sees the ad, or comparing the same group before versus after a campaign. GORDON: We have research we did with Facebook that looks at this. We were wondering if companies could rely on statistical models using obser - vational data, i.e., not data generated from an experiment but simply data collected in the normal course of running a campaign. Our question was: How well do these observational methods do? And the beauty of partner - ing with Facebook was they let us directly compare randomized exper - iments with several observational approaches to assess whether they were a good substitute. And by and large, we found that unfortunately Based on insights from Brett Gordon
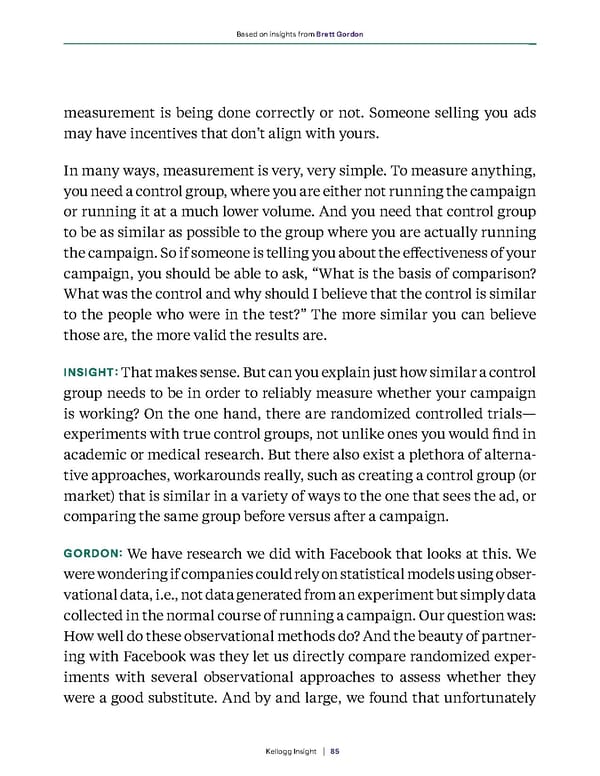